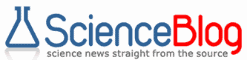
1997 From: Human Factors and Ergonomics Society
Pilots Who Rely Too Much On Automation May Not Detect Malfunctions Robert Molloy and Raja Parasuraman, Catholic University of America, Washington, D.C.ABSTRACT The present study examined the effects of task complexity and time on task on the monitoring of a single automation failure during performance of a complex flight simulation task involving tracking, fuel management, and engine-status monitoring. Two groups of participants performed either all three flight simulation tasks simultaneously (multicomplex task) or the monitoring task alone (single-complex task); a third group performed a simple visual vigilance task (simple task). For the multicomplex task, monitoring for a single failure of automation control was poorer than when participants monitored engine malfunctions under manual control. Furthermore, more participants detected the automation failure in the first 10 min of a 30-min session than in the last 10 min of the session, for both the simple and the multicomplex task. Participants in the single-complex condition detected the automation failure equally well in both periods. The results support previous findings of inefficiency in monitoring automation and show that automation-related monitoring inefficiency occurs even when there is a single automation failure. Implications for theories of vigilance and automation design are discussed. Requests for reprints should be sent to Raja Parasuraman, Cognitive Science Laboratory, Catholic University of America, Washington, DC 20064. INTRODUCTION Automation is ubiquitous in many modern work settings, but perhaps most so in aviation. Automation in aviation, as in other domains, has increased demands on the pilot to monitor systems for possible failures. As research on vigilance has shown, this is a role for which humans are poorly suited (Davies & Parasuraman, 1982; Parasuraman, 1987; Wiener, 1987). Additionally, pilot overreliance on automation can make detecting failures more problematic under certain conditions (Mosier, Skitka, & Korte, 1994). Pilots using automation may ignore other sources of information that can signal an automation failure. For example, in 1979, Eastern Flight 401 crashed into the Florida Everglades when the crew failed to detect the autopilot disengaging and did not monitor altitude because they were engaged in a possible problem with the landing gear (National Transportation Safety Board [NTSB], 1973). In 1985, China Airways Flight 006 plummeted 31 000 feet when the crew, preoccupied with an engine problem, did not notice the autopilot gradually losing control of the plane (NTSB, 1986). In both these incidents, the crew (a) was at the end of a long shift, (b) was highly qualified, (c) should have detected the occurrence given the salience of the event, (d) were preoccupied with another task, and (e) may have overrelied on automated systems. Problems in monitoring automated systems are further evident in pilots' reports of incidents. Mosier et al. (1994) examined NASA's Aviation Safety Reporting System (ASRS) database and found that 77% of the incidents in which overreliance on automation was suspected involved a probable vigilance failure. The vast majority of incidents occurred during cruise, when the pilot's primary role was to monitor and supervise the automation. Similarly, Gerbert and Kemmler (1986) studied German aviators' anonymous responses to questionnaires about automation-related incidents and also reported the largest contributor to human error to be failures of vigilance. Despite the wealth of incident and survey data, relatively few empirical or controlled studies of automated systems in aviation tasks have been performed (Kessel & Wickens, 1982; Parasuraman, Molloy, & Singh, 1993; Thackray & Touchstone, 1989; see Satchell, 1993, for a general review of cockpit monitoring). Kessel and Wickens (1982) examined the effects of automation on monitoring for participants performing a two-dimensional (2D) pursuit-tracking task. Participants who manually controlled the tracking task detected subtle changes in the system dynamics more readily than did participants who passively monitored automated tracking. Thackray and Touchstone (1989) examined the effect of automation on performance of a simulated air traffic control task. Participants monitored a radar display for two aircraft on the same flight path at the same altitude. Half the participants were provided an automated aid for detecting the conflicts, whereas the rest had no aid. Twice during a 2-h session, early and late, the automated aid failed. Although aided participants were slower at responding to conflicts the first time the aid failed than were unaided participants, response times for the aided group the second time the aid failed were equivalent to those of the unaided group. Thackray and Touchstone concluded that their results did not support the view that automation impairs monitoring performance. They may have failed to find evidence of poor monitoring of automation because their participants performed only one task, a situation rare in the cockpit. As ASRS incident reports suggest (Billings, Lauber, Funkhauser, Lyman, & Huff, 1976; Mosier et al., 1994), many monitoring failures occur when the pilot is engaged in multiple tasks. Parasuraman and colleagues (1993) therefore reasoned that monitoring of automation would be poor only under multiple-task conditions. They tested nonpilot participants on a laboratory flight simulation task consisting of 2D compensatory tracking, probability monitoring of engine status, and fuel management. In the multiple-task condition, participants performed the tracking and fuel management tasks manually, and an automation routine detected and fixed engine malfunctions. In the single-task condition, participants had only to "back up" the automated engine status task. The automation routine would fail from time to time. Participants were responsible for detecting these failures and for making the appropriate response to fix the malfunction. Although participants normally had a detection rate of over 70% when performing the engine status task manually (a baseline condition), their detection rate substantially declined when performing the task with the automation routine in the multitask condition. However, Parasuraman et al. (1993) found that when engine monitoring was the only task, detection was equally accurate ( 100%) and about as quick ( 2.5 s) during manual performance as under automated control. The Parasuraman et al. study (1993) is recognized as providing the first empirical evidence, in a controlled setting, of poor monitoring resulting from an overreliance on or excessive trust in automation (Lee & Moray, 1992; Riley, 1994). However, one criticism of this study is that the automation failure rates were artificially high (e.g., 12%) and unlikely to be representative of any real automated system (or at least one that would be used by human operators). Therefore, the question arises whether monitoring of automation is inefficient in a more realistic setting, in which only a few automation failures occur or even a single failure occurs. Examining monitoring performance with a single automation failure also raises an enduring issue in vigilance research--that is, whether the vigilance decrement over time for detecting critical signals occurs when only one such signal is presented to the participant (Davies & Parasuraman, 1982; Loeb & Binford, 1970; Warm, 1984). Poor monitoring of automation has been linked to lowered vigilance (Billings et al., 1976). However, Parasuraman et al. (1993) did not find evidence for a vigilance decrement over time in the detection rate of automation failures. One factor could be the relative complexity of the flight simulation task in the Parasuraman et al. (1993) study: Participants had to monitor up to four gauges showing different engine parameters while simultaneously performing manual tracking and fuel management tasks. There is some evidence, though not unequivocal, that the vigilance decrement is reduced or absent when participants have to monitor several displays in a complex task, as opposed to when they monitor a single display, even though the overall level of performance may be lower (Parasuraman, 1986). For example, Howell, Johnston, and Goldstein (1966) found that performance on a complex monitoring task was associated with lapses rather than with an overall decrement over time. A second factor could be the relatively high signal rates used. Participants received anywhere from 6 to 16 automation failures (signals) per 30-min session in the Parasuraman et al. (1993) study. High signal rates increase the detection rate in simple vigilance tasks and may also moderate the extent of the vigilance decrement (Davies & Parasuraman, 1982). Therefore, the question arises: Is there a vigilance decrement when the participant has to monitor for only a single signal? Loeb and Binford (1970) conducted one of the few vigilance studies in which participants received only one signal per session. Participants were required to detect a single critical event--a noise pulse that was 1.8 dB louder than 70 dB nonsignal noise pulses. Participants completed five 1-h sessions in which the signal appeared at a different time within each session. Significantly fewer participants detected the signal when it occurred in the fourth and fifth 12-min blocks than when it was presented in the first and second 12-min blocks of the 1-h watch. The often-repeated criticism that vigilance research is of little practical import because of the artificially high signal rates used in laboratory studies (Mackie, 1984, 1987) is diluted by Loeb and Binford's important results. However, despite its importance to the elucidation of vigilance behavior, few attempts have been made to verify Loeb and Binford's finding, particularly for visual displays. Accordingly, one of the goals of the present study was to examine the efficiency of human monitoring of automation when only a single failure occurs. Such a finding would considerably bolster the evidence in favor of the view that highly reliable automated systems can engender poor monitoring behavior. A second goal was to examine the vigilance decrement as a function of task complexity. Therefore, in the present study we repeated Loeb and Binford's study using a simple visual vigilance task rather than the auditory task they used. In addition, vigilance effects were also examined for the complex multiple-task flight simulation used by Parasuraman et al. (1993). Finally, to examine the impact of task complexity, we also investigated a single-task version of the flight simulation. The following predictions were made. First, participants performing the complex flight simulation task would have a low probability of detecting the single automation failure, following Parasuraman et al. (1993). It was also expected that participants monitoring a simple visual discrimination task and those performing the multiple-complex task would show a vigilance decrement in detecting the automation failure (signal), thereby replicating Loeb and Binford's (1970) findings and demonstrating that a vigilance decrement can occur in complex automated tasks with realistically low signal rates. Finally, participants in the single-task version of the flight simulation task were expected to detect the automation failure, following Parasuraman et al. (1993). METHODS Participants Participating in this study were 36 student volunteers (20 men and 16 women) who received compensation of $15 each. The volunteers ranged in age from 18 to 38 years, were right-handed, and had normal (20/20) or corrected-to-normal vision. All 36 students had some computer experience; a majority (28) used a computer at least once a week. In addition, 33 students had experience using a joystick. Tasks Multicomplex task. We used a revised version of the Multi-Attribute Task Battery (MAT; Comstock & Arnegard, 1992) developed by Parasuraman, Bahri, and Molloy (1991). This multitask flight simulation package, which consists of tracking, monitoring, and fuel management tasks, was used because (a) it has some similarity to actual flight-deck functions; (b) the component tasks are dynamic, adding to the sense of realism of the task; (c) the tasks can be automated; (d) the degree of automation reliability can easily be changed; and (e) the number of tasks can be manipulated. The descriptions of the three tasks are given next. System monitoring task. The monitoring task consisted of four vertical gauges with moving pointers. The gauges were marked as indicating the temperature (TEMP1, TEMP2) and pressure (PRES1, PRES2) of the two aircraft engines. In the normal condition, the pointers fluctuated in a pseudo-random manner around the centers of each gauge within one scale marker in each direction from center. The mean fluctuation rate was different for each gauge (0.244 Hz, 0.128 Hz, 0.294 Hz, and 0.159 Hz for the four gauges). Independently and at intervals according to the preprogrammed script, each gauge's pointer shifted its "center" position away from the middle of the vertical gauge to one scale marker above or below its center. This shift could be detected by noting that the pointer was drifting beyond one scale marker either above or below the center of the gauge. The participant was responsible for detecting this shift, regardless of its direction, and responding by pressing the corresponding function key (T1, T2, P1, or P2). The appropriate response key was identified below each vertical display. Following a correct response, the pointer of the scale to which the participant responded immediately moved back to the center point and remained there without fluctuating for a period of 1.5 s. If the participant failed to detect a shift within 10 s, the shift was reset and the participant was credited with a miss. If a participant pressed a response key and no gauge had shifted its center position, the participant was credited with a false alarm. When the task was automated, a red warning light came on following an offset, signaling that the automation routine had detected the problem. Four seconds after the offset, the red light went off and the offset pointer returned to normal. During these automation resets the keyboard was disabled. An automation failure occurred when an offset was not accompanied by the red warning light. If an automation failure was not detected within 10 s, the gauge would reset and the participant was credited with a miss. As will be described further, the automation failed only once during a 30-min session. The performance measures for the system monitoring task were the percentage of participants detecting the automation failure, speed of detection, and number of false alarms. Tracking task. A first-order, 2D compensatory tracking task with joystick control was presented in the top center portion of the display. Participants were required to keep a green circular cursor as close as possible to a crosshair located in the center of the display window. The cursor moved in x and y directions according to a specified forcing function consisting of a sum of nonharmonic sine waves. The highest frequency of the forcing function was 0.06 Hz in this study. The control dynamics were first-order, or velocity control, provided by a displacement joystick. Operator performance of the tracking task was evaluated by sampling the x and y control inputs at 10 Hz and thus deriving the x and y deviations. The x and y deviations were combined to yield a composite deviation measure. The root mean square (RMS) error was then computed for the samples obtained over a 1-s period. RMS error scores for successive 1-s intervals were averaged over a longer period of performance (e.g., 15 min) to yield a mean RMS error score for a block. Fuel management task. This task simulated the actions needed to regulate fuel in the aircraft. The task was presented below the tracking task (see Figure 1). The display consisted of six tanks (rectangles) connected by a series of pumps (lines). Participants were required to maintain a specified level in the main tanks, A and B. Both of the main tanks were depleted at a constant rate, simulating fuel usage. In order to complete their task, participants had to transfer fuel from the lower supply tanks to the main tanks. They toggled specific pumps on and off by pressing a corresponding key on the keyboard. When activated, a pump moved fuel from one tank to another at a rate indicated under the pump status window and a direction indicated by the arrow next to the pump. The performance measure for this task was mean RMS error in the fuel levels of the main tanks. RMS errors (from the required 2500 gallon [9462.5 liter] level) were obtained every 30 s. The deviation scores for both tanks were combined, and composite RMS scores were computed for each 15-min block of the session. Single-complex task. In this task only the system monitoring window from the MAT was used; the tracking and fuel management windows were blanked out. As in the multicomplex task, an automation routine detected and reset engine faults, but the automation failed on a single occasion. Participants were required only to monitor the automation. Performance measures were the percentage of participants detecting the automation failure, speed of detection, and false alarms. Simple task. The simple task was a visual discrimination task requiring successive discrimination of squares that differed in size (Parasuraman and Mouloua, 1987). In the task a series of squares were presented on the center of the screen (for 700 ms) at a rate of one per second. Participants were required to press the space bar to respond to a nonstandard (signal) square. The standard or nonsignal square was 3.2 cm and the signal square was 2.7 cm. A single critical signal, which represented an automation failure, was presented during the session. The performance measures for the task were the percentage of participants detecting the automation failure, speed of detection, and false alarms. Apparatus All tasks in the experiment were controlled by a 386AT computer running at 33 MHz. The display for the task was a 13- inch VGA color monitor with a dot pitch of 0.29 and a scan rate of 60 Hz. Tracking input was controlled by a Quickshot Warrior V joystick. Procedure Participants were randomly assigned to one of the three task conditions. The experimenter orally instructed participants, who subsequently completed a 5-min training block during which they received eight critical signals (or automation failures). Because previous vigilance research has shown that probability-contrast effects occur when participants receive higher signal rates during training than during the main vigilance session (Colquhoun & Baddeley, 1967), participants received a second 5-min training block in which only a single critical signal occurred. They were told prior to this block that the occurrence of a signal in this session would be rare. At the end of both training blocks, on-screen performance feedback was given to each participant. After a 5-min break, participants received instructions on the main task. Those in the two complex-task groups were told that an automation routine would detect engine parameters that went out of range and would automatically reset them; participants in the simple group were told that an automation routine would prevent the small square from appearing on the screen. They were instructed to oversee the automation routine and, if it failed, to respond to the signal by pressing the appropriate response keys. In one session the automation routine failed within the first 10 min of the 30-min session, whereas in the other session, the failure occurred during the last 10 min. Within each 10-min block, the failure, if it occurred, did so at the same time for all participants. Participants performed both sessions with the order of time of occurrence of signals (early or late) counterbalanced across sessions and participants. Participants received no feedback at the end of the first session and received a 10-min rest period between sessions. RESULTS The results for the two practice blocks are shown in Tables 1 and 2. In both blocks participants performed the monitoring task under manual control; the only difference between blocks was that only a single failure occurred in the second block. Participants were highly accurate in detecting this single signal (automation failure) in the second block; no participants missed the signal in the single-complex task, and only two (of 24) missed it in the other two task conditions. In addition, participants in the simple task condition generally responded more quickly and made more false alarms than did those in the other conditions. Because there were no order effects for any of the dependent measures, the two main 30-min test sessions were combined for analysis. Because of the low data rates (i.e., only one signal per session), a nonparametric statistical test, Wilcoxon's T, was used to test the significance of differences in detection rate and reaction time (RT) between the first and the last block. False alarm changes over time were analyzed using t tests. Monitoring performance under automation control was first examined by comparing the detection rate in the 30-min test session with that in the second practice block. (The data from the first practice block were not analyzed because of the higher signal rate in that block.) Significantly more participants detected the single signal in the manual practice block than in the automated test session for both the simple task group (83% vs. 42%) and the multicomplex group (83% vs. 58%). There was no significant difference for the single-complex task (100% vs. 88%). Figure 2 shows the mean percentage of automation failures detected across participants in each group. Each point represents the proportion of participants in that group who detected the automation failure. For the simple visual discrimination task, there was evidence of a vigilance decrement; detection of the single critical signal was better in the first 10 min of a session than in the last 10 min. Participants assigned to the multicomplex group showed a similar pattern. However, participants assigned to the single-complex task showed no evidence of a vigilance decrement. Overall detection performance was also efficient in this group, with most participants detecting both the early and late automation failures. False alarms are plotted in Figure 3. Only the simple task showed a significant block effect for false alarms. The number of false alarms emitted in this task increased over time. RTs for detection can be seen in Figure 4. Both complex groups showed an approximately 1-s increase in RT over time. However, these differences were not statistically significant. There were no significant block effects for either tracking RMS error or fuel management RMS error in the multicomplex task. Performance on both tasks remained stable over time. DISCUSSION The results supported the three main predictions of this study. First, participants performing the complex flight simulation task were less efficient in monitoring for a single failure in an engine status task under automation control than when they monitored under manual control. Second, a vigilance decrement in detecting the automation failure (signal) over time was found both for this task and for a simple visual discrimination task. Finally, when participants monitored automation under single-task conditions, without performing other manual tasks, monitoring performance was highly accurate. Many anecdotal and survey reports have suggested that human operator monitoring of automation is poor under particular conditions that can occur in the aircraft and other work environments (Mosier et al., 1994; Wiener, 1988). Nevertheless, empirical evidence under controlled conditions for monitoring inefficiency was relatively scant until a study by Parasuraman et al. (1993), in which monitoring of an engine status task was compared under manual and automated control. The automation failure rate was relatively high in that study, certainly higher than would reasonably be encountered in any real system. The results of the present study replicate the findings of Parasuraman et al. (1993). More important, they show that human monitoring of automation is also inefficient when only a single failure of the automation occurs, if the operator is engaged in other manual tasks, as is likely to occur on occasion in the flight deck or control tower. Monitoring of the engine status task under automated control was markedly poorer than was monitoring when the task was performed manually. These findings boost the evidence in favor of the view that highly reliable automated systems can engender poor monitoring performance, as originally suggested by Billings et al. (1976), probably because of overreliance on or excessive trust in automation (Mosier et al., 1994; Riley, 1994). The present study found that a vigilance decrement can occur in the performance of complex automated tasks. Furthermore, a vigilance decrement was also observed for a simple visual discrimination task, thereby replicating the results of Loeb and Binford (1970), who first showed that vigilance declines even when participants have to monitor for only a single critical auditory signal. The results imply that theories of vigilance that have been formulated with respect to multiple signal presentations (Davies & Parasuraman, 1982) may also apply to the single-signal case. Moreover, the results rule out one of the original theories of the vigilance decrement, Mackworth's (1950) inhibition theory. Mackworth theorized that the vigilance decrement was similar to the extinction of a conditioned response that was no longer reinforced: Participants made voluntary conditioned responses to signals but were not reinforced for their response; hence their rate of responding decreased. Loeb and Binford's (1970) study brought into question Mackworth's inhibition theory because, with only one signal, response extinction prior to the signal could not occur. Yet, because Loeb and Binford used an auditory task, the theory could still have applied for visual vigilance tasks. In the present study a vigilance decrement was found for a task with a single visual signal. Moreover, participants showed an increase in response rate, as evidenced by a significant increase in false alarms over time rather than response extinction. The results thus provide evidence against Mackworth's hypothesis. The finding that a vigilance decrement was found for both the simple visual task and the multicomplex task but not the single-complex task is suggestive of the applicability of the well-known inverted-U relationship. The evidence in favor of the inverted-U relationship between arousal (task complexity) and vigilance performance is not compelling (Davies & Parasuraman, 1982; Hancock & Warm, 1989). Nevertheless, in this study the results indicate that the relationship between task complexity and the presence or absence of a vigilance decrement may be an inverted-U relationship, as was suggested by Wiener, Curry, and Faustina (1984). Although participants completing the more complex system monitoring task did not show evidence of a vigilance decrement compared with participants completing the simple visual discrimination task, vigilance did decline when the system monitoring task was made more complex by adding tracking and resource management. This result supports the claim that giving a person too much or too little to do can result in reduced vigilance (see Weiner, Curry, & Faustina, 1984). The results of the present study have implications for many of the most often voiced criticisms of laboratory vigilance research. Mackie (1987) criticized much of vigilance research for having unrealistically high signal rates that do not reflect so- called real-world tasks. The present study used a signal rate of a single signal per session. The rate may still be lower for certain critical events found in real aviation environments (about once every two weeks for a wide variety of industrial and military monitoring jobs, as estimated by Craig, 1984). Nevertheless, one signal per session represents a very low rate compared with most other studies of this kind. The evidence suggests that the vigilance decrement would actually be more pronounced for rates lower than this (Davies & Parasuraman, 1982). Thus one would expect a greater decrement in real settings in which signal probability is likely to be very low. A second criticism of vigilance research argues that the vigilance decrement is only a laboratory phenomenon found with simple visual and auditory displays (Adams, 1987; Kibler, 1965; Mackie, 1984, 1987). However, task complexity in and of itself cannot explain the occurrence of a vigilance decrement (Parasuraman, 1986, 1987). The present study found evidence for a decrement when the engine status monitoring task was performed simultaneously with two other tasks but not when it was performed alone. Additionally, evidence of a vigilance decrement in field studies of actual complex monitoring jobs (or very close simulations of such tasks) have been reported in a few instances (see the review in Parasuraman, 1986). A recent example is a study by Donderi (1994) of shipboard search performance, in which a vigilance decrement over time was found. There are some limitations to the current study. First, although the majority of the participants had computer and joystick experience, none had any prior experience with the experimental tasks. Even though research on vigilance has found decrements using both unpracticed volunteers and experienced operators (Davies & Parasuraman, 1982), replicating this research with experienced pilots in a simulator would be desirable. Second, the length of the vigil in this experiment was only 30 min. This task length of vigil may be shorter than the duration of sustained operations in some real-world settings. However, Nachreiner (1977), in an analysis of several operational monitoring tasks, found that uninterrupted observations of greater than 20 min occurred very infrequently. Moreover, previous studies show that if a vigilance decrement occurs in a task, whether simple or complex, it is typically complete by 30 min, with little or no deterioration beyond that time (Davies & Parasuraman, 1982). The present results have some implications for aviation automation. At the most general level, they show that human monitoring of automation, though often effective, can be poor under certain conditions--for example, when operators are engaged simultaneously in other manual tasks. Second, this study provides the first empirical evidence of a vigilance decrement for automation monitoring. Researchers have previously suggested that such problems could arise (Wiener, 1985), but their claims have sometimes been dismissed as arising from research based on unrealistic and simplistic tasks. Irrespective of whether a vigilance decrement occurred or not, however, the finding that operator monitoring of automation can be poor suggests the need for additional training or the use of other countermeasures against pilot overreliance on automation. Airbus Industries, recognizing this problem, has begun a training program that tests pilots with several types of automation anomalies. An important issue for further work is to determine to what extent automation training can mitigate some of the performance costs associated with poor operator monitoring of automation. Robert Molloy is a Transportantion Research Analyst with the National Transportation Safety Board. He received his Ph.D. in psychology in 1996 from Catholic University of America, Washington D.C. Raja Parasuraman is professor of psychology and director of the Cognitive Science Laboratory at the Catholic University of America in Washington D.C. He received a Ph.D. in psychology in 1976 from the University of Aston, Birmingham, England. [Extracted from HUMAN FACTORS: THE JOURNAL OF THE HUMAN FACTORS AND ERGONOMICS SOCIETY, Vol. 38, No. 2, June 1996. Copyright 1996 by the Human Factors and Ergonomics Society, P.O. Box 1369, Santa Monica, CA 90406-1369 USA; 310/394-1811, fax 310/394-2410, [email protected], http://hfes.org. Contact Lois Smith to obtain a copy of the full article, including references, tables, and figures.]
| |